Original HGFI Hainan Green Finance Research Institute included in the collection #ESG data 1 #ESG indicator 1 #ESG disclosure 1 # rating company 1 # investment decision 1.
Wen | Ji Yufei
Words in this article: 7222 words
Reading time: 15 minutes
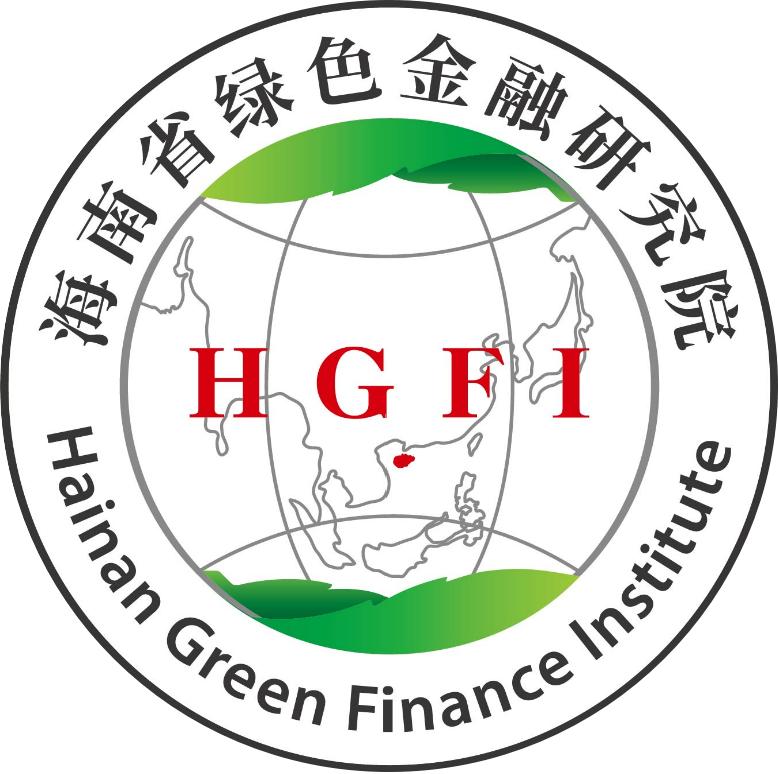
catalogue
First, there is a huge difference in ESG rating between domestic and foreign rating companies.
(1) ESG ratings of international rating companies vary greatly.
(2) ESG ratings of domestic rating companies are equally different.
Second, the current data problems in ESG evaluation
(1) The original data is inconsistent.
(2) reference datum selection is arbitrary.
(3) The estimation of replacement value is uncertain.
Iii. suggestions
(1) Enterprises should continuously report comparable ESG data.
(2) Investors should promote enterprises to disclose relevant ESG indicators.
(3) The Exchange shall formulate mandatory requirements or guidelines for ESG disclosure.
(4) Data suppliers should disclose their data processing methods as much as possible.
And reach an industry consensus
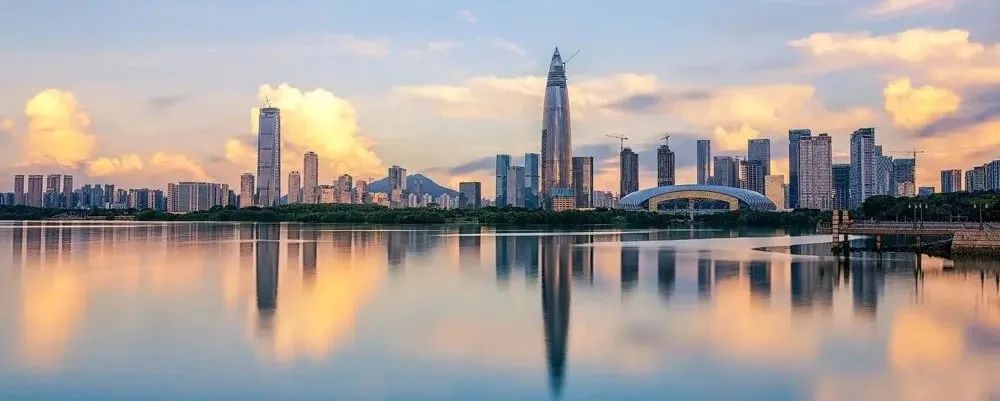
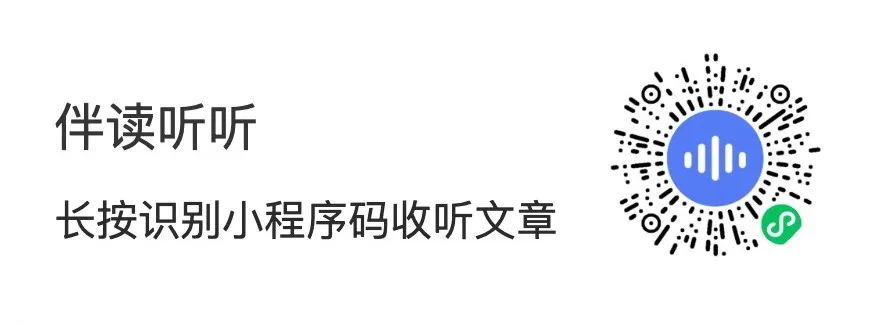
In recent years, ESG rating, as the basis of value judgment to guide investors’ investment decisions, has been paid more and more attention by international investors, and domestic investors have also begun to pay attention to ESG information disclosure and rating. However, the ESG data collected by rating companies, especially the methods of data processing, are quite different, and the ESG rating results of different rating companies are also very different, which seriously affects the use of ESG rating by investors. As the most important input to support the function of ESG indicator system, the way of processing data will directly affect the scoring results of related indicators. This paper intends to do some exploration and research on the data processing in ESG evaluation.
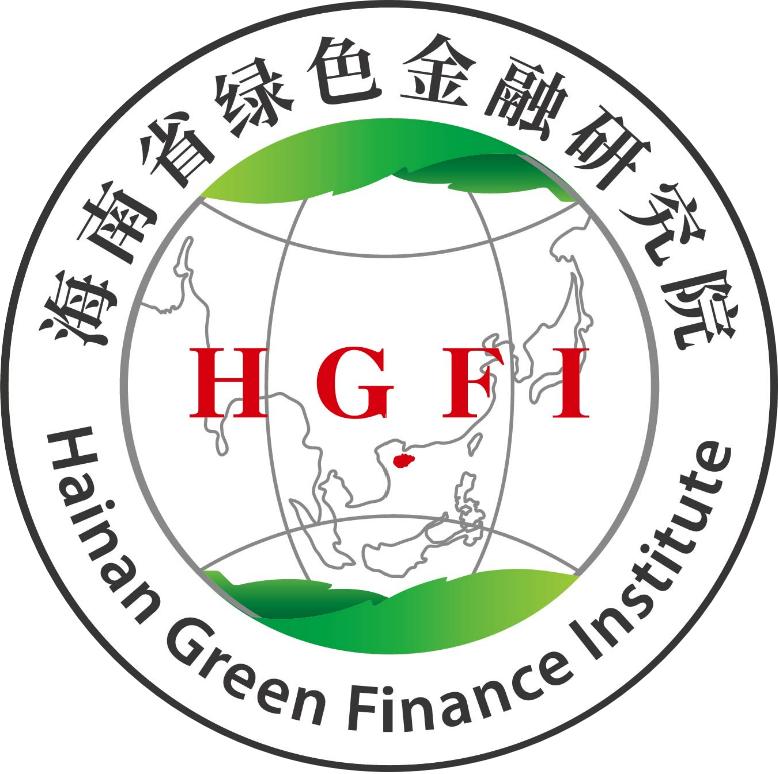
1. There is a huge difference in ESG rating between domestic and foreign rating companies.
The rating results of ESG by different rating companies are very different at home and abroad.
(A) ESG ratings of international rating companies vary greatly.
Billio et al.(2021) studied and compared the ESG rating results of MSCI (morgan stanley capital international), Refinitiv (Luft), Sustainability Analytics (a product of Morningstar) and RobecoSAM (the data source of Dow Jones Sustainability Index [1]), and found that the average correlation between these rating results was only 0.58 (lowest 0.43, highest 0.69). The average probability of coincidence between these rating results is only 24% (minimum 19%, maximum 28%) [2], and the low correlation and low coincidence probability of rating results [3] mean that the rating results of different rating companies are very different.
The following table shows the ESG rating results of Nissan Motor Co., Ltd, Verizon Communications Inc, Oracle Bone Inscriptions Corp. and Goodman Group by four rating companies, and the differences are obvious. Among them, the rating range of Refinitiv, Sustainalytics and RobecoSAM is 0 to 100, while the rating range of MSCI is from CCC to AAA.
Scoring results of different international ESG rating companies (2021)
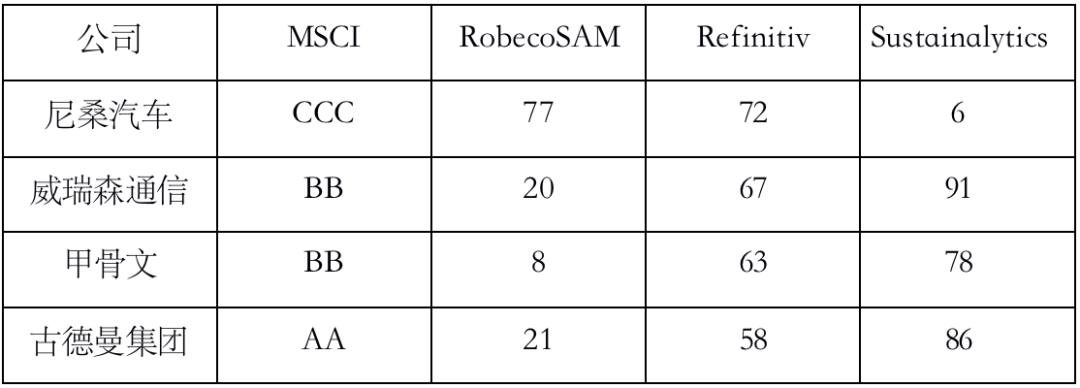
Source: Billio et al.(2021)
This huge rating difference will make market investors feel at a loss, and it is difficult to make investment value judgments and investment decisions.
(B) ESG ratings of domestic rating companies are equally different.
There are many domestic ESG rating companies, including Wonder, Weizhong Lanyue, Jiashi, China Securities Index, Dingli Corporate Governance, Green Business, China Securities Carbon Neutralization, Social Value Investment Alliance and so on. According to Wonder’s data, four domestic listed companies, China Resources Micro, China Petroleum, Shengli Precision and China Pharmaceutical, are randomly selected. Through comparison, we can find the differences between the ratings: Wonder gives China Resources Micro AA rating, while Weizhong only gives B rating; Social Value Investment Alliance gives China Petroleum AA- rating, while Shangdao Ronglv only gives B+ rating; Huazheng Carbon Neutralization gives Shengli Precision a ranking of 65/4040, while Harvest only gives a ranking of 3478/4569. FTSE Russell gave China Pharmaceutical a 33/76 industry ranking, while Dingli Corporate Governance only gave a 59/79 industry ranking.
Scoring results of different domestic ESG rating companies on China Resources Micro.
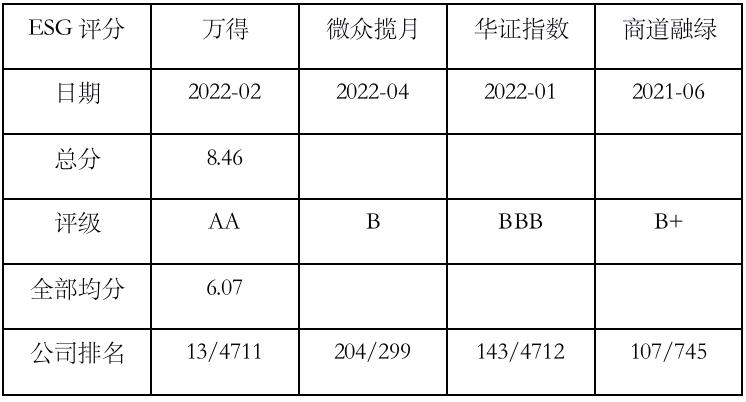
Source: Wonder
Scoring results of China Petroleum by different domestic ESG rating companies.
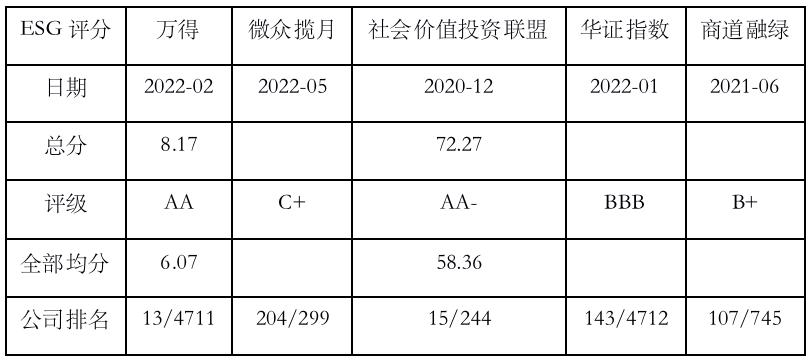
Source: Wonder
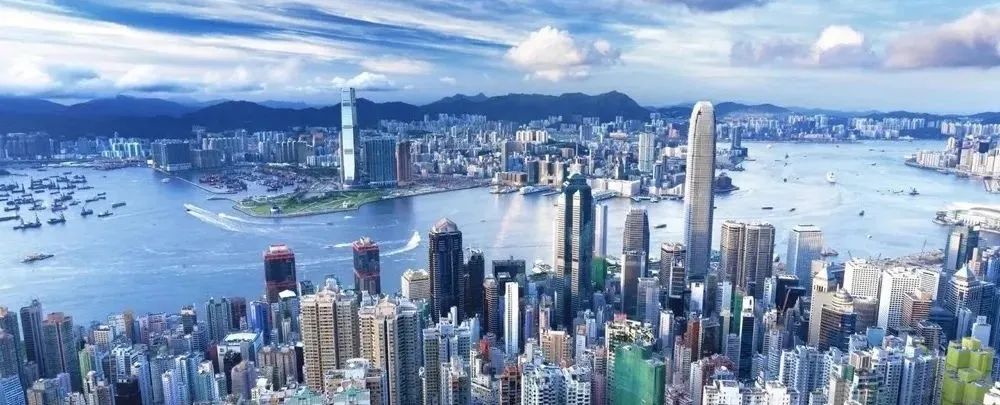
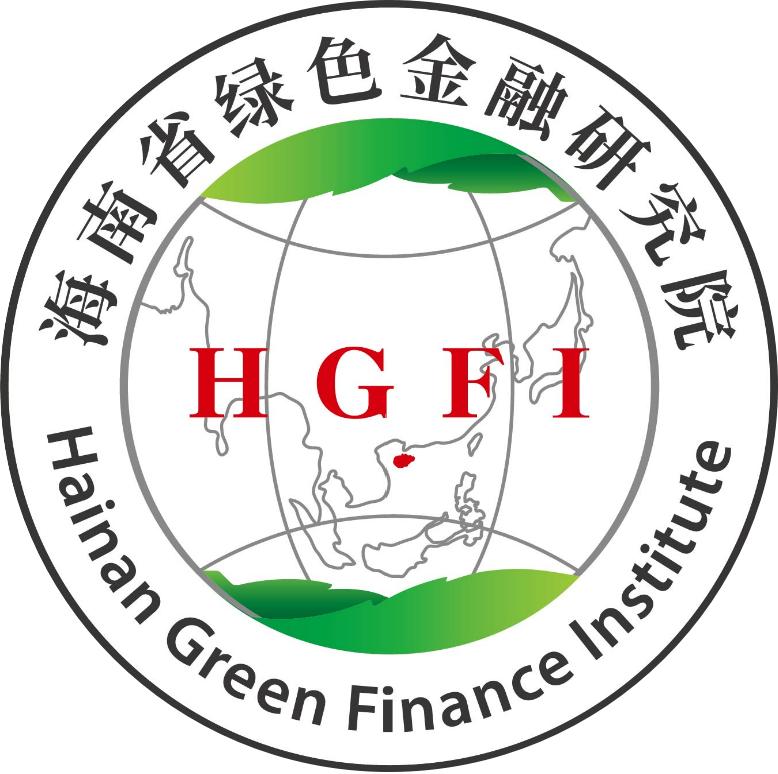
Second, the current data problems in ESG evaluation
The ESG ratings of rating companies to enterprises are very different. On the one hand, the goals and values, design principles of rating system and selection rules of indicators of rating companies are different, which leads to different rating results. On the other hand, the data problem is also an important reason for the huge difference in the results of different rating companies. Different rating companies have different channels and quality of obtaining data and different methods of processing data, which will also bring great differences in rating results.
In the current ESG rating, in terms of data acquisition and processing, there are some problems to be solved, such as poor consistency of original data, arbitrary selection of reference standards, and uncertain replacement process of lost values.
(A) the original data is highly inconsistent
The "inconsistency" here does not emphasize the difference of information sources, but emphasizes that the multi-dimensional attributes of the original data samples (such as specific wording, measurement angle, data unit and other formal attributes, as well as statistical attributes such as average, standard deviation and extreme value) are very different.
Rating companies generally collect the original ESG data from the annual sustainable development report or ESG report disclosed by enterprises every year. However, the consistency of relevant data in the annual sustainable development reports or ESG reports of different enterprises is very low. Taking the topic of "labor health and safety" as an example, Kotsantonis and Serafeim(2019) randomly selected the sustainable development reports of 50 large listed companies in Fortune 500 (Fortune 500), and collected the information manually by the author. The indicators used to describe the topic of "labor health and safety" are as follows: lost time (frequency), lost time (accident rate per 100 people, 5,000 people, and 200,000 people), injuries that lead to lost time, accident rate, accidents that require vacations, days lost due to injuries, financial losses due to accidents, injuries that lead to more than one day lost, injury rate, and days lost rate. Time loss of less than 61 days caused by occupational illness or injury, time lost (serious accident rate), number of accidents, number of accidents without time loss, number of lost working days, number of serious accidents, injury rate per 200,000 hours of work, reduction of working days caused by work-related injuries, claimed time lost, time lost, number of accidents of occupational diseases, occupational illness rate, occupational illness rate and occupational disease rate.
Faced with such diverse data forms, it is difficult to determine which indicator is the best indicator to measure the performance of enterprises on the topic of "labor health and safety". Moreover, the units of these indicators are not the same, including no units, ratios, percentages and other different units. Most importantly, the statistical distribution characteristics (mean, standard deviation, extreme value, etc.) of different indicators are obviously different, which makes it very difficult to compare and integrate data across indicators [4].
The real problem is that when enterprises disclose the performance of a certain topic, they often only choose the most favorable indicators for their own enterprises to disclose. Rating companies (data providers) can’t obtain multiple indicators for the same enterprise, but in the face of various indicators provided by many enterprises, each rating company (data provider) has its own processing methods, and the inconsistency of the original data disclosed by each enterprise ultimately lies in the differences in the integration process of the original data of each rating company. The core problem here is that there is the data of the enterprise before the rating standard, and the defects of the data itself will naturally be left in the rating results [5].
(B) reference benchmark selection is arbitrary.
Even if there is no difference in the original data, different ESG rating companies have different scores on the same performance of the same enterprise. One of the important reasons is that the selection of scoring reference benchmarks is very arbitrary. Setting up different "reference benchmarks", that is, double standards or flexible standards, is an effective means to systematically change the scoring results for the same (same type) enterprise.
Rating companies can choose to evaluate all enterprises under a unified reference standard; Enterprises can also be classified according to the characteristics of industry and country, and multiple parallel reference benchmarks can be set up, and only enterprises belonging to the same subclass can be evaluated under their corresponding reference benchmarks.
Using a unified reference frame, the scoring results are comparable across industries, but the scoring results inevitably produce industry bias. Taking environmental issues as an example, the performance of the oil and gas industry will naturally be lower than that of commercial banks. If only some enterprises are evaluated under the same reference standard, the industry deviation of the scoring results is small, but the cross-industry comparability of the scoring results is correspondingly weakened. Worse than reducing cross-category comparability, the process of classifying different enterprises and selecting reference benchmarks is not transparent. Rating companies can move the relative position of the same enterprise in the distribution corresponding to different reference benchmarks by freely selecting reference benchmarks [6], thus indirectly affecting the scoring results.
In addition, how to deal with diversified enterprises will also be a problem to be solved.
(C) The estimation of replacement value is uncertain.
The range of data required for ESG evaluation is very wide. For a specific enterprise, all the original data required for rating are often not disclosed (or cannot be provided), and missing values often appear in samples. The missing value needs to be estimated and replaced by the estimated result (replacement value), and then the evaluation process continues. At present, rating companies and data providers do not have a transparent, unified and reliable methodology to estimate the replacement value of the unavailable ESG data (that is, the lost value).
The uncertainty of the estimation process of replacement value is divided into two levels: first, there are many alternative estimation methods, and the replacement values (estimation results) given by different methods are not consistent, and each company has not fully disclosed the selection of estimation methods, accordingly, investors have no way to evaluate and interpret the estimation process and rating results of each company; Second, even if each company chooses the "optimal" estimation method, the accuracy of the estimation itself is limited by the model characteristics and statistical laws. Under the circumstances that the correlation process is difficult to model, the correlation between variables is poor, the sample size is limited, and the continuity of the estimated value is low, the estimation process cannot be guaranteed to be accurate, and the rating result using the replacement value (estimation result) cannot be guaranteed to be accurate. The first level of uncertainty comes from the disclosure choices of rating companies and data providers (that is, the estimation process is not transparent and unified), and the second level of uncertainty comes from the estimation method itself (that is, the estimation process is not reliable enough). At present, the estimation methods of replacement value (replacement method) include rule-based replacement method, input-output model replacement method, statistical replacement method and so on, and the scenarios in which these estimation methods can operate reliably and effectively are very limited.
The uncertainty of rule-based substitution method comes from the process of setting rules. The accuracy of scoring results of this substitution method depends on the precise setting of rules, and the rule-makers need a lot of industry knowledge as support. Some rules will even affect the disclosure of relevant data by enterprises, further increasing the uncertainty of data acquisition. Taking the indicator of "the number of deaths in the workplace" as an example, the rating company can set a rule that "if there is no data source, the number of deaths in the workplace is assumed to be zero". According to this rule, the scoring results will be systematically superior to the actual performance of enterprises, which also encourages enterprises to refuse to disclose relevant data in disguise (especially for those small and medium-sized enterprises with high information disclosure costs).
The replacement method of input-output model is difficult to estimate the data related to social responsibility, because compared with the laws of natural science, the laws of interaction between people are more complicated and uncertain. Therefore, the input-output model is generally used to estimate the environment-related indicators of some industries, such as estimating the output variables such as carbon dioxide emissions with input variables such as coal consumption and electricity consumption. The accuracy of the input-output model replacement method depends on the extent to which the model system can reproduce the actual behavior process of the enterprise. In the estimation of environment-related indicators in some specific industries, the consumption of raw materials and related macroeconomic variables of enterprises are easy to obtain, and the use mode and output results of raw materials are also highly deterministic in objective scientific laws. When the above conditions are met, the replacement method of input-output model can give a highly accurate estimation.
Statistical replacement method generally needs regression analysis [7], and its accuracy is limited by the availability of regression prediction input variables, the correlation between regression prediction input variables and predicted values and the continuity of predicted values. The transparency of correlation prediction (regression) process is very low, and the public can’t determine to what extent the prediction methods used by rating companies and data providers meet the requirements of statistical laws.
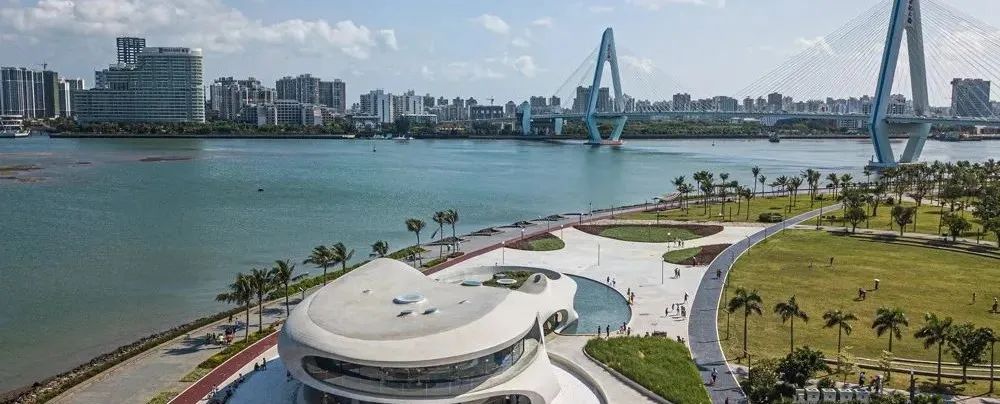
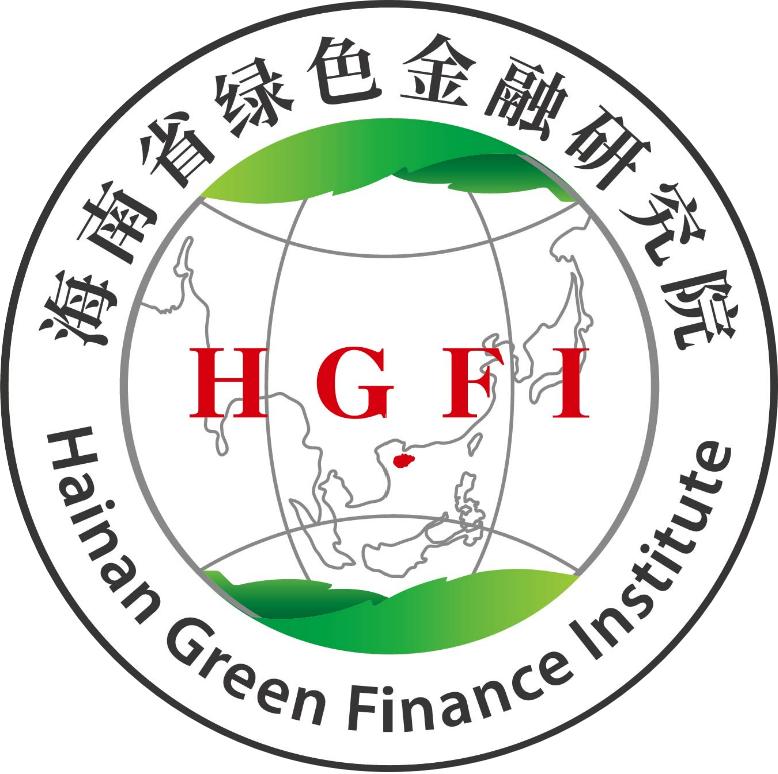
III. Recommendations
To solve the problems of low disclosure degree and consistency of ESG data, high arbitrariness in the selection of scoring criteria and replacement value estimation methods, it needs the joint efforts of many market participants. Here are some suggestions from the perspective of enterprises, investors, exchanges and data providers, so as to better achieve the sustainable development goals through ESG rating.
(A) enterprises should continue to report comparable ESG data.
The ESG data provided by different enterprises are very different, which involves the influence and restriction of many factors such as the market, industry, management and risks faced by enterprises. Therefore, it is particularly important for individual enterprises to choose a suitable ESG disclosure standard, and continue to disclose ESG data according to this standard, so as to maximize the comparability (or consistency, that is, the unification of data forms in the time dimension) of the data. Enterprises should be strictly self-disciplined and regularly disclose ESG data and information in a fixed format (such as tabular form similar to financial statements) in accordance with relevant regulatory requirements. Enterprises need to adjust the corporate governance structure and set up a special department to manage ESG-related risks and related data disclosure.
Global Reporting Initiative (GRI) and International Financial Reporting Standards Foundation (IFRS Foundation) have provided their own sustainable disclosure standards. The latter emphasizes the concerns of investors as stakeholders and uses money as a unified measure to maintain the consistency of financial statements, while the former tries to balance the concerns of wider stakeholders and give more independent recognition to the importance of different indicators. Enterprises should choose their own sustainable disclosure standards according to their own development, and qualified enterprises can also take the initiative to join higher standards of international standards to obtain international comparability.
Although the disclosure of ESG data will bring additional costs, enterprises should see that actively disclosing high-quality ESG data is an important means to attract investors and maintain their own good social image, which is beneficial to the long-term development of enterprises.
(B) Investors should promote enterprises to disclose relevant ESG indicators.
At present, although enterprises provide a large amount of ESG data and information, it is difficult for investors to make investment decisions, risk judgments or early warnings by using these incomparable data. Therefore, when investors push enterprises to disclose relevant ESG information, they should put forward clear and comparable data requirements for enterprises. For example, when an investor invests and finances an enterprise, it should be clearly stated that ESG information is also information that has a significant impact on the value of the enterprise’s securities and needs to be disclosed immediately. The enterprise should disclose ESG information together with financial information in the offer and invitation to offer and other documents (such as debt prospectus and prospectus). For enterprises that fail to fulfill their obligation to disclose ESG information, investors can also try to safeguard their own interests by legal means and promote the improvement of relevant legislation.
(3) The Exchange shall formulate mandatory requirements or guidelines for ESG disclosure.
The establishment of ESG disclosure guidelines or even mandatory requirements by the exchange can enhance the transparency of related behaviors of enterprises and help enterprises maintain good investor relations. From the practice of various exchanges, the requirements for ESG information disclosure of listed companies have become higher and higher.
In 2012, HKEx issued the ESG Report Guidelines as a voluntary disclosure proposal for listed companies. From 2016, some suggestions will be raised to the level of semi-mandatory disclosure, and the "explain without disclosure" rule will be implemented. In May 2019, HKEx issued a consultation document on the revised ESG Reporting Guidelines, and in December of the same year, the contents of the new ESG Reporting Guidelines were determined, further expanding the scope of mandatory disclosure, comprehensively adjusting the disclosure proposal to "explain without disclosure" and continuously improving the requirements for ESG information disclosure of listed companies.
China Securities Regulatory Commission has also begun to require listed companies to disclose ESG information, but it is still in its infancy. In February, 2021, CSRC issued the Guidelines on Investor Relations Management of Listed Companies (Draft for Comment), requiring the inclusion of environmental protection, social responsibility and corporate governance (ESG) information. In June, 2021, CSRC issued Contents and Format of Annual Report and Contents and Format of Semi-annual Report, adding Section V Environmental and Social Responsibility. In April, 2022, China Securities Regulatory Commission issued "Guidelines for the Management of Investor Relations in Listed Companies", which took environmental, social and governance (ESG) information as one of the contents of communication between listed companies and investors. However, there is still a lack of normative and consistent guidance for specific operations. It is suggested to formulate specific rules in these aspects and put forward requirements for disclosing specific data. The disclosure requirements of ESG data by external constraints of companies such as exchanges and investors can fundamentally solve the problems of high inconsistency of original data and high probability of missing values, thus avoiding the problem of "data comes first, standards come first".
(4) Data suppliers should disclose their data processing methods as much as possible and reach an industry consensus.
At present, the scoring methodology of ESG data providers at home and abroad is very opaque, so it is not enough to provide a list of important topics. Data providers need to describe in more detail how they define the benchmark of statistical comparison and how to deal with the key process of building rating indicators such as real data and substitute data, otherwise, the credibility of relevant ratings will be impossible for all stakeholders. On this basis, data providers should fully communicate and reach as broad a methodological consensus as possible in practice (such as reaching a consensus on the selection of reference benchmarks and replacement value estimation methods), thus reducing the randomness of ESG rating results and enhancing the readability of rating results.
Although the disclosure of data processing methods is beneficial to all stakeholders, it is not in the self-interest of data providers, and data processing methods will not be disclosed voluntarily. Data processing method is the core knowledge of data suppliers. Once it is made public, competitors in the market can quickly master it, and the market value of its data will also decline rapidly. Therefore, in order to ensure full competition and information disclosure in the data supply market, all stakeholders should support the establishment of more data suppliers who are willing to disclose their data processing methods to join the market competition. The highly transparent data processing methods respond to the demands of all stakeholders, so they are also highly competitive in the data supply market. Finally, through the full competition in the data supply market, existing data suppliers are forced to disclose their data processing methods more.
Data providers usually collect ESG information required for rating periodically every year, and the specific methods include: company questionnaire; Analyze company documents (such as sustainable development report); Interview individuals, company employees and other stakeholders (such as trade unions, NGOs, etc.); Use Natural Language Processing (a branch of artificial intelligence and linguistics) and other artificial intelligence technologies to mine unstructured data (such as TruValue Labs). By 2018, more than 100 organizations are collecting and analyzing ESG data and providing ESG ratings [8].
Data providers use the collected data to construct various indicators and sell their indicator data, although their methodology of how to construct indicators using raw data is not transparent to the public. In addition, many organizations use the secondary data [9] provided by ESG data providers to build their own ratings and rankings, and provide comprehensive index [10] solutions. Bloomberg and Thomson Reuters (Thomson Reuters) are the mainstream ESG data platforms used by these organizations, which often include other broader financial information such as securities prices.
It can be seen that data suppliers should ensure the transparency and reliability of data collection and processing, which is also a key prerequisite for ESG rating companies to accurately evaluate the relevant performance of enterprises.
Quote footnote:
[1] Some organizations that use enterprise questionnaires to collect ESG data do not sell their own data. RobecoSAM is a sustainable investment asset management company, managing assets worth $120 billion (in 2018), and inviting more than 3,400 companies to fill out questionnaires every year. These ESG data are used as the basis for investment decisions within the company and used to construct the Dow Jones Sustainability Indices (DJSI). CDP (formerly known as Carbon Disclosure Project), a non-governmental organization, also collected data related to environmental risks of about 6,400 companies through questionnaires. The design of the questionnaires referred to the framework of the Task Force on Climate-related Financial Disclosures (TCFD).
[2] The data of the rating company comes from Refinitiv (Luft, one of the providers of financial market data and infrastructure, formerly known as the Finance and Risk Department of Thomson Reuters. After independence, Blackstone Group acquired 55% equity and Thomson Reuters acquired 45% equity. ) financial terminal Eikon/DataStream, Bloomberg financial terminal and MSCI (morgan stanley capital international) database.
[3] The correlation here can be understood as whether any two rating companies have the same rating judgment on the overall sample composed of several enterprises, such as: A rating company scores 50 points for enterprise A, 60 points for enterprise B and 70 points for enterprise C; The rating company B scores 60 points for enterprise A, 70 points for enterprise B and 80 points for enterprise C, so the ratings of the whole sample {A, B and C} by the two rating companies A and B are different, but they are highly correlated (completely related). The variable describing the difference of a rating result itself is fit or fit probability, for example, A rating company scores 50 points for enterprise A, 60 points for enterprise B and 70 points for enterprise C; If the rating company B scores 50 points for enterprise A, 70 points for enterprise B and 80 points for enterprise C, then the two rating companies A and B only give the same score for enterprise A, and the rating fit for the whole sample {A, B and C} is 33.33% or 1/3.
[4] The first two differences can be understood as differences in the formal attributes of a single point of data, and the third difference is not about the attributes of a single point of data, but about the statistical attributes of the whole sample. For example, for a certain topic (a), the samples of six companies {A, B, C, D, E, F} are scored, and three companies {A, B, C} are selected in the annual report. Suppose that the data of {A, B and C} on indicator (a1) are {100, 200 and 300} respectively, while the data of {D, E and F} on indicator (a2) are (100, 4000 and 10000) respectively, the difficulty for rating companies is how to determine {A}. This problem can be solved by statistical analysis to some extent. The solution of statistical analysis requires the following assumptions: First, indicators (a1) and (a2) can truly and accurately reflect the performance of the whole sample in topic (A) and have the same statistical distribution characteristics; Second, the sample is rich enough to restore the common true statistical distribution characteristics of (a1) and (a2) respectively through the statistical distribution characteristics of the whole sample. For example, there are 2,000 enterprises in the sample instead of six.Moreover, the (a1) statistical distribution of the 1,000 enterprises using the (a1) indicator and the (a2) statistical distribution of the 1,000 enterprises using the (a2) indicator are both normal distributions. According to statistical analysis, it can be inferred that the enterprise that ranks x% (where X is in the range of 0 to 100) among the 1,000 enterprises using the (a2) indicator is also ranked X.
[5] Similarly, there are three ways to measure Covid-19 infection: antigen, nucleic acid and antibody, and the effectiveness of products provided by companies under each category is different. Assuming that in a national screening, everyone only provides the results of one COVID-19 infection measurement method, then it is difficult for us to give equal ratings to antigen-positive, nucleic acid-positive and antibody-positive. This is because, limited by the objective laws of measurement methods, the true statistical distribution of the three measurement results is different for the same sample (the same screening population).
[6] According to the assumption at the beginning of this paragraph, there is no difference in the original data, and both rating companies of Party A and Party B have obtained all samples {A, B, C, D, E, F} and the index scores of six enterprises on a certain topic {100,200,300,700,800,900}. Both rating companies of Party A and Party B use the following rating rules. If they reach the set reference benchmark, they will be rated as excellent; if they fail to reach the set reference benchmark, they will be rated as poor. Among them, the A-rated company chooses the average value of all samples as the reference benchmark, that is, 500. Then the scores of {A, B and C} three energy enterprises failed to meet the standards, while the scores of {D, E and F} three commercial banks were all higher than the standards, and the results of rating company A for six enterprises were {inferior, inferior, inferior, excellent, excellent}. The rating company B sets the reference benchmark according to the industry average, and the rating results of the rating company B for the energy industry and the banking industry are {poor, excellent and excellent} and {poor, excellent and excellent} respectively. Give an example to illustrate the meaning of this sentence, that is, the C-rated company can choose to transfer from the reference benchmark of A-rated company to the reference benchmark of B-rated company, thus realizing that: the energy enterprises of {B} and {C} have moved from the last 50% of the overall rating distribution to the first 66% of the new internal rating distribution of the energy industry; D commercial banks have moved from the top 50% of the overall rating distribution to the bottom 33% of the new banking internal rating distribution.
[7] Both the regression replacement method and the prediction mean matching replacement method need to determine the replacement value through regression analysis. The difference is that the regression replacement method directly replaces the missing value with the prediction mean; The matching replacement rule of predicted mean replaces the missing value with the actual observed value in the sample closest to the predicted mean, which is a partial parameter method.
[8] According to the data of the Global Initiative for Sustainable Rating (GISR) GISR)2018. Some organizations providing ESG data are profit-making and some are non-profit; Some focus on topics such as climate change or human rights, while others focus on topics of all ESG categories. ESG data providers continue to improve the richness of their services, from selling data and research to providing consulting services and technical and management solutions. At the same time, ESG data providers continue to seek to expand and internationalize the number of companies in their databases.
[9] ESG data can be divided into three levels according to the degree of data processing: the original data collected by data providers can be defined as the lowest level ESG data; The original data is processed by the data provider into secondary ESG indicators (data); According to their own rating methodology, ESG rating companies finally integrate many secondary ESG index data into a tertiary ESG rating (data).
[10] For example, AMI(Access to Medicine Index) uses the data of Sustainalytics, while FTSE4Good Index Series (FTSE 4 Good Index) uses the research service of Vigeo-EIRIS.
References:
Billio, M., Costola, M., Hristova, I., Latino, C., & Pelizzon, L. (2021). Inside the ESG Ratings:(Dis) agreement and performance. Corporate Social Responsibility and Environmental Management, 28(5), 1426-1445.
Eccles, R. G., & Stroehle, J. C. (2018). Exploring social origins in the construction of ESG measures. Available at SSRN 3212685.
Kotsantonis, S., & Serafeim, G. (2019). Four things no one will tell you about ESG data. Journal of Applied Corporate Finance, 31(2), 50-58.
Original title: Data Problems and Suggestions of Industry Research | |ESG Evaluation
Read the original text